Method
Academic Research Method
The Global University Alliance (GUA) is an open group of academics (professors, readers, lecturers and researchers worldwide) with the ambition to provide both industry and academia with state-of-the-art insights into research and artefact design. The importance of research methods and design concepts within both academia and industry is not a new phenomenon. Knowledge exchange between these two parties is both mutually beneficial, as well as continuous, bi-direction and symmetric in the sense that although often different in nature contributions by practitioners should be valued as much as academic contributions to the knowledge base.
As work everywhere becomes more collaborative, the need to develop concepts for the analysis and development of collaborative research and design between academia and industry is identified. This paper therefore, aims at presenting the knowledge gap in existing research and design methods and introduces a framework for analysing and developing Collaborative Research and Design between Academia and Industry”. When academics build artefacts for practitioners, these artefacts need to be constructed rigorously to meet academic standards and need to be relevant for practitioners (von Rosing, Laurier, 2015). Construction rigour is typically considered to be the domain of academia, while practitioners have been acknowledged to create knowledge and artefacts relevant to themselves and others (Nonaka, Umemoto, & Senoo, 1996).
As a revolutionized way of working between academia and industry, the Global University Alliance promotes an innovative way of thinking, working and modelling by taking full advantage of a mutually beneficial collaboration between academic research and industry design through evaluation by practitioner through application of GUA artefacts in the real world. The GUA’s structured way of working is based on both construction rigor and practical relevance of concepts and artefacts. To manage the size and complexity of the research topics addressed and to promote networking across universities, lecturers and researchers, the GUA has defined research responsibilities in key areas. In each of these key areas, research coordinators were appointed.
An example of a collaborative Industry Academic Design their names and industry organizations can be found in this document. They are a blend of academics, standard bodies, governments and industry experts. The foundational thinking of this Industry Academic Design, is the key research responsible’s task to provide an international platform where universities and thought leaders can interact to conduct research on the key aspects of the overall research.
Many hundreds of people (academics and practitioners) have been directly involved over the many years in researching, comparing, identifying patterns, peer reviewing, categorizing and classifying, again peer reviewing, developing models and meta models, again peer reviewing, and at last but not least developing standards and reference content with industry. Through this iterative peer review process that involves both academics and practitioners as reviewers and contributors. As illustrated in figure 1, they do this through defining clear research themes, with detailed research questions, where they analyse and study patterns, describe concepts with their findings.
This again can lead to additional research questions/themes as well as development of artefacts which can be used as reference content by practitioners and industry as a whole. What the GUA also does uniquely is the collaboration with standards bodies like:
- ISO: The International Organization for Standardization.
- CEN: The European Committee for Standardization (CEN).
- IEEE: The Institute of Electrical and Electronics Engineers is the largest association of technical professionals with more than 400,000 members.
- OMG: Object Management Group: Develops the software standards.
- NATO: North Atlantic Treaty Organizations (NATO’s) with the 28 member states across North America and Europe and the additional 37 countries participate in NATO’s Partnership for Peace and dialogue programmes, NATO represents the biggest non-standard body that standardises concepts across 65 countries.
- ISF: The Information Security Forum, Investigates and defined information security standards.
- W3C: World Wide Web Consortium-The W3C purpose is to lead the World Wide Web to its full potential by developing protocols and guidelines that ensure the long-term growth of the Web/Internet.
- LEAD: LEADing Practice – the largest enterprise standards body (in member numbers), which actually has been founded by the GUA. The LEADing Practice Enterprise Standards are the result of both the GUA research and years of international industry expert consensus and feedback on the artefacts and thereby repeatable patterns.
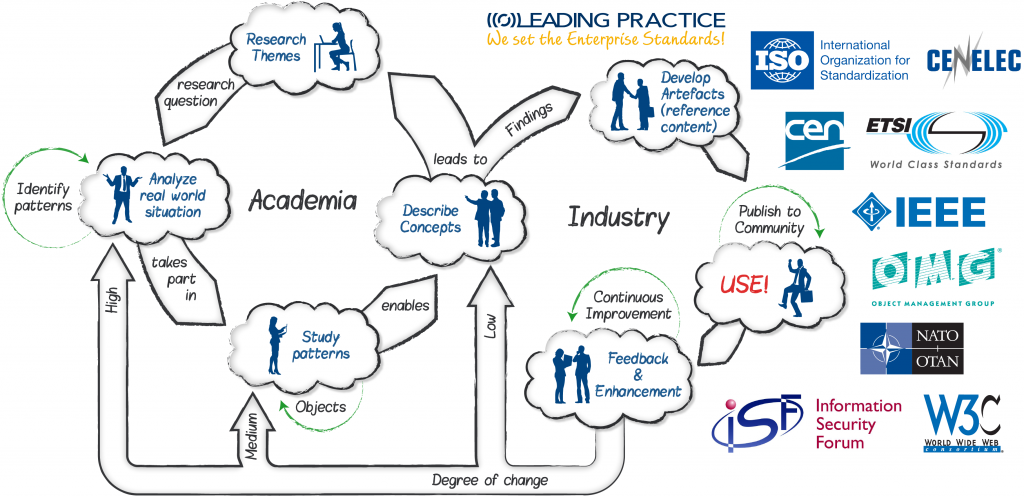
The Academic & Industry Approaches
We in the GUA do not only work with other standards developing organizations like ISO, IEEE, OMG, OASIS, and NATO etc., but also work with various industry organizations and standards setting organizations (governments, NGOs, etc.). Among some of them are the US Government, the Canadian Government, the German Government, and others. Most relevant is that the academia and industry process used in the Global University Alliance and the various collaborative industry practitioners has two types of different cycles.
As is illustrated in figure 2, the one where Academia is leading the research and innovation, this is called the Academia Industry Research (AIR) process. The other is where practitioners from Industry describe concepts and develop artefacts and thereby they bring about innovation. This process is called the Academia Industry Design (AID).
Our research of the GUA way of working concludes that the Academia Industry Design interlink between academia and practitioners in the following ways:
- Academia defines:
- At the Abstraction level the typical setup is that Academia typically designs the research themes with research questions and thereby the solutions at the type level (concepts and solution for a type of problem).
- The knowledge creation processes in terms of analyzing real world situation and patterns as well as studying patterns interlinks between rigor and relevance, of which the rigor aspect can be analyzed in theory best and the relevance can be tested in practice best.
- Thereby, combining explicit knowledge to develop new explicit knowledge. Academia typically combines explicit knowledge at type or instance level to create new knowledge concepts at type level.
- Industry Practitioners:
- Typically design solutions/artefacts at instance level (solution for a particular problem).
- Combine explicit knowledge at type or instance level to create new knowledge at instance level. Thereby creating an ideal interaction and loop between academia and industry practitioner around research themes and research questions.
The internalization, socialization as well as externalization happens in interaction between both the academic and industry practitioners in the following ways:
- Internalization: Converting explicit knowledge (e.g. books, standards) to tacit knowledge (e.g. personal knowledge). Academia typically teaches explicit knowledge to be transformed into tacit knowledge of students (e.g. practitioners). Whereas practitioners typically study academic concepts and non-academic solutions to develop competencies (tacit knowledge).
- Socialization: Sharing tacit knowledge through interaction. Academia research share tacit knowledge in doing research and publications together. Whereas practitioners share tacit knowledge by doing things together (and learning from each other while doing).
- Externalization: The need to convert tacit knowledge into explicit knowledge. Academia study in this context, what practitioners do (at instance level) to create new knowledge at type level. Whereas practitioners sometimes document what they do, and sometimes share this content (e.g. industry standards, best practices).
- Combination: This is where internalization, socialization as well as externalization applies combined with academia and industry.
Building unique knowledge with the Academia Industry concept
Applying the AIR and AID arrangement in the GUA over 15 years has facilitated the acquiring and building of a unique set of knowledge on patterns and practices in the industrial world. Already after 5 years of working in the AID setup, in 2004 the GUA started to formally represent their knowledge as a set of concepts within a domain, and the relationships between those concepts.
The GUA chose to use the concept of ontology as their basis for categorizing and classifying all their concepts (von Rosing & Laurier). It thereby provides the basis for both a shared vocabulary and the very definition of its objects and concepts. It is quite common to use the notion of ontology for the categorization as well as classification of concepts, both in academia(Gomez-Perez et al. 2004; von Rosing & Laurier, 2016; Borgo 2007, Lassila and McGuinness 2001; etc) as well as in industry OWL, OMG, MOF, Zachman Enterprise Ontology etc.
Each of them have a specific purpose, therefore the categorization and the classification is focused on the expressivity and formality of the specific languages used/proposed: natural language, formal language, etc. The other more general applicable categorization as well as classifications of the ontologies, is centred around the scope of the objects described by the ontology. (Roussey, C., Pinet, F., Ah Kang, M., and Corcho, O. 2011).
Since the enterprise ontology of the Global University Alliance, is and should be generally applicable within any organization. The more general applicable categorization and classifications of the ontologies, was chosen. Thereby the Ontology classification is centered around the sphere, filed and level and the categories is grounded on the scope of the objects described by the ontology.
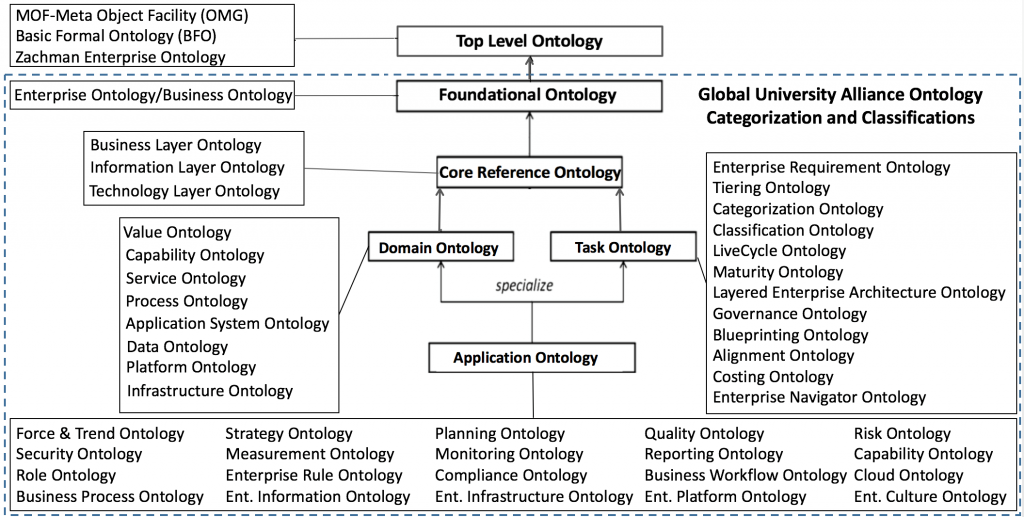
The GUA, has found that there is a benefit of categorizing and classifying the ontologies around the scope of the objects described. For instance, the scope of an application ontology is narrower than the scope of a domain ontology; domain ontologies have more specific concepts than core reference ontologies, which contains the fundamental concept of a domain. Foundational ontologies can be viewed as meta ontologies that describe the upper level concepts or primitives used to define the other ontologies. (Roussey, C., Pinet, F., Ah Kang, M., and Corcho, O. 2011, von Rosing, Zachman 2017).
We use MOF-Meta Object Facility (OMG), Basic Formal Ontology (BFO) and Zachman Enterprise Ontology as some of our Top-Level Ontologies. The Top-Level Ontology describes primitives that allow for defining very general concepts like space, time, matter, object, event, action, etc. (Adapted from N. Guarino, 1997) Provides the foundation for the formal system that allows for developing meta-meta-models, of which the completeness and clarity needs to be guaranteed trough a mapping between a top-level ontology and the formal system’s primitives (MOF). (von Rosing, Zachman 2017). Using for example MOF to structure the academic research by the various industry design artefacts is found in figure 4.
The Enterprise/Business Ontology is the Foundational Ontology. It is a generic ontologies applicable to various domains. It defines basic notions like objects, relations, structure, arrangements and so on. All consistent ontology should have a foundational ontology. (Roussey et al, 2011) Foundational ontology can be compared to the meta model of a conceptual schema (Fonseca et al. 2003). It is a system of meta-level categories that commits to a specific initial-view. We use the foundational ontology, to provide real-word semantics for general conceptual modelling languages, and to constrain the possible interpretations of their modelling primitives. As such, we map our meta-meta-model (M3) to our foundational ontology. Both to certify its comprehensiveness and clarity. It also ensures that all can and will relate through our Enterprise/Business Ontology.
The Business Layer Ontology, Information Layer Ontology and the Technology Layer Ontology are our Core Reference Ontologies. They are the standard used by all our different groups of users. These type of ontology are linked to a specific topic/domain but it integrates different levels and tiers related to specific group of users. We know from theory that core reference ontologies as well as domain ontologies based on the same foundational ontology can be more easily integrated. (Roussey et al, 2011).
Our layered enterprise ontologies are the result of the integration of the sublayer domain ontologies. However, they are a formal (i.e., domain independent) system of categories and their ties that can be used to construct models of various domains, and not one of a specific domain. Our core reference ontologies are built to catch the central concepts and relations of the specific layers. They provide the foundations for a (generic) modelling language trough a mapping between the core reference ontology and the modelling language’s meta-model (M2).
The Domain Ontologies of Value, Competency, Service, Process, Application, Data, Platform and Infrastructure, describe, the context and vocabulary related to their specific domain by specializing the concepts introduced in the core-reference ontology. In the Enterprise/Business Ontology, the domain ontologies are linked to a specific core reference ontology layer. In terms of the MOF tiers, they provide the foundations for a domain-specific modelling languages (M2) trough a mapping between the domain ontology and the modelling language’s meta-model. Each specific domain ontology is only valid to a layer with their specific view point, however the layers relate through the semantic relations, captured in the foundational ontlogy. Therefore, the individual viewpoints, ensures the ability to engineer, architect or model across multiple sublayers. That is to say that the viewpoints defines how a group of users conceptualize and visualize some specific phenomenon of the sublayers. The domain ontologies could be linked to a specific application. (Roussey et al, 2011) They provide the foundations for a domain-specific modelling languages (M2) trough a mapping between the domain ontology and the modelling language’s meta-model. (G. Guizzardi, 2005).
The Tiering Ontology, Categorization Ontology, Classification Ontology, LiveCycle Ontology, Maturity Ontology, Governance Ontology, Blueprinting Ontology, Enterprise Requirement Ontology as well as Layered Enterprise Architecture Ontology are all a part of the Task Ontologies. They provide the basis to the generic tasks relevant to both the domain ontologies and application ontologies. They do this by specializing the terms introduced in the core-reference ontology, therefore ensuring full interoperability across the various task ontologies and the core reference, domain and the application ontologies. The task ontology contains objects and descriptions of how to achieve a specific task, on the other hand the domain ontology portrays and defines the objects where the task is applied. In terms of the MOF tiers, they provide the foundation for a task-specific modelling language (M2) trough a mapping between the task ontology and the modelling language’s meta-model.
The Application Ontologies describe concepts of the domain and task ontologies. Often the Application Ontologies are specializations of both the related ontologies in order to fulfil the specific purpose of a specific use, function, purpose and thereby application. In terms of the MOF tiers, they provide the foundation for a model (M1) trough a mapping between the application ontology and the model.
The Global University Alliance has the following Application Ontologies:
- Force & Trend Ontology
- Strategy Ontology
- Planning Ontology
- Quality Ontology
- Risk Ontology
- Security Ontology
- Measurement Ontology
- Monitoring Ontology
- Reporting Ontology
- Capability Ontology
- Role Ontology
- Enterprise Rule Ontology
- Compliance Ontology
- Business Workflow Ontology
- Cloud Ontology
- Business Process Ontology
- Information Ontology
- Infrastructure Ontology
- Platform Ontology
- Enterprise Culture Ontology
Academic Research: Identification of Repeatable Patterns across Industry Design concepts
As a part of the 2004 detailed academic research, which was the foundation of developing the Enterprise Ontology, we identified the most common meta objects, stereotypes, types and subtypes with all their definitions and over 10.000 semantic relationships that were common across all organizations, business units, departments and agencies.
There were plenty surprises along the way, one of them was that despite being independent of size, product or service when the objects existed within the organization, they had the same semantic relationship. It surprised us, because were these findings really true? We analyzed 10 different industry sectors, namely the Financial Services, Industrial Sector, Consumer Packaged Goods, Energy, Public Services, Healthcare, Utilities, Transportation, Communication and the High Tech sector organizations with the same output and results. The semantic relations were the same. Even when analyzing and researching the 52 sub-industries we came to the same conclusion.
While certain industries had specific meta objects with types and subtypes relevant for their industry, all the industries had the meta objects listed in publication “Using the Business Ontology to develop Enterprise Standards” (von Rosing, 2017). All the industries also had the same semantic relations. The findings led to a lot of questions in our research team, so we decided to analyze what differentiated the organizations in their way of working with the objects.
In order to understand the behavior, we decided to examine the activities of the industry leaders (financial outperformers in each industry). We examined data from the Standard & Poor’s archives during a period from 1994 to 2004, and later again from 2004 to 2014. As part of the GUA research, we scrutinized the differences between the responses of financial outperformers and those of underperformers over a 10 year period. For organizations with publicly available financial information, we compared revenue and profit track records with the average track records for those in the same industry.
We analyzed and cross-referenced the findings to other existing research that have proven that there is a connection to organizations approaches and their overall performance (Malone, T.W., Weill, P., Lai, K, D’Urso, V., Herman, G., Apel, T., Woerner, S., 2006). MIT (Malone, 2004), Accenture Research (Accenture, 2009), IBM Institute for Business Value (IBM, 2008, 2009, 2010, 2011, 2012), Business Week Research (BW, 2006), and The Economist Intelligence Unit (Economist, 2009)(Economist, 2009), 2010, 2011, 2012)pendent authors that this would be worthy of further investigation. Especially, since throughout the analysis, there was gathered information and conclusions, based on these top- and bottom-half groupings of the organizations that outsmarted and outcompeted their peers.
The analysis confirmed that the outperformers and underperformers both had the objects identified as well as the same semantic relations. But there was a difference between how the outperformers versus the underperformers worked with the objects. We identified that the outperformers did the following, which the underperformers consistently didn’t do.
They identified which objects were:
- Important to develop the core differentiating aspect of the organization to outthink, outsmart and outcompete other organizations. The outperformers converge on the revenue model and value model to strengthen the competitive advantage with emphasis on innovation. It was less than 5% of the organization that was core differentiating in terms of adding to the value model and the revenue model. The objects relevant to the core differentiating aspects are the foundation for design thinking and innovation.
- Relevant for core competitiveness. Contrary to general thinking, it was less than 15% of any organization’s aspects that was relevant for the core competitiveness, and thereby head to head industry competition of the organization. The outperformers focused on performance model and service model to improve the competitive parity with emphasis on efficiency, innovation and transformation.
- Significant for the non-core aspects of the organization. In the organizations analyzed, it was more than 80% of the organization that was non-core, and thereby do not add to the differentiation or competitiveness of the organization. In those areas the outperformers focused on the cost model and operating model to standardize, harmonize, align, optimize and thereby enabling cost cutting.
A notable difference was that the underperformers in general didn’t identify their core differentiating, their core competitive nor their non-core aspects. So while they worked with the relevant objects, such as identifying the disruptive industry forces and trends, developed their enterprise strategy, specified their critical success factors etc., they did not realize that the concepts they applied them to needed different ways of working and modelling.
In figure 4, we have illustrated the patterns that we identified. Exemplifying the connections between the business context researched and the repeatable patterns identified (i.e. best practices, industry practices and/or leading practices). Additionally, how the patterns should be automated within the technology perspective.
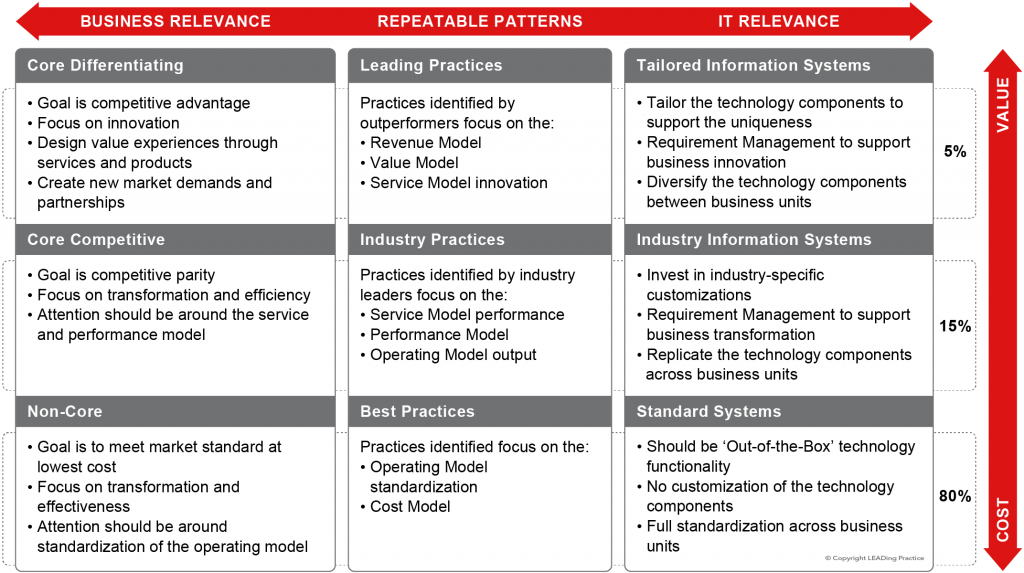
There were multiple repeatable patterns identified, both in the business, information and technology layer. Below are some examples of repeatable patterns identified:
Business Layer:
- Disruptive forces and trends that can influence the core differentiating aspects of the enterprise. The patterns are therefore Leading Practices that help to outperform, outsmart and outcompete the competition. The patterns were identified in 51 different industries.
- Benchmarks on which strategies are being used for the core differentiating, the competitive as well as the non-core aspects. The strategies were distinctive for the core differentiating aspects versus the non-core aspects.
- Most critical organizational capabilities – those that are the basis for both core differentiating and core competitiveness (across 51 different industries).
- Integrated planning (typical functions, processes, KPIs, and the flows involved as well as the continuous improvement loops).
- Most common non-core capabilities and processes across organizations, such as Finance, HR, IT, Procurement, etc. This enables organizations to reuse the content as well as to help them standardize and cut cost.
- Industry-specific processes that helps organizations develop their core competitive performance model as well as help standardize the operating model.
- Critical KPIs (across 51 different industries) that help organizations in their reporting, control and decision making activities.
Information Layer:
- Most common SAP blueprints, both in terms of processes automated in SAP modules, application tasks as well as the SAP system measures. What is relevant is that the level of tailoring and customizing these ERP systems is mostly way too high (and often done in the wrong places). The tailoring of the information systems should only happen within the core differentiating aspects of the organization. While important, is procurement, HR or finance etc. really the core differentiating components within the organization? While it obviously depends on the industry, products and services, the most likely reason you need to standardize it is to improve the operating model and reduce cost. Consequently, huge customizations do not add value, but most likely enforces your unique way of working, where you are nonetheless not unique (and neither should be). The standardization is important in some areas, but should be done with out of the box functionality (i.e. software vendor best practice).
- Most common Oracle blueprints, both in terms of processes automated in Oracle workflows, modules, application tasks as well as the Oracle system measures.
- Most common way of calculating the information system performance measures. These findings were quite important for analytics, business intelligence, reporting and decision making.
- And many others.
Having made these astonishing findings, in 2004, we decided in the GUA to both work with the existing standards bodies, such as ISO, CEN, IEEE, NATO, UN, OMG etc., as well as to create an enterprise standard body (LEADing Practice) that develops the enterprise standards and the patterns. Packaging the patterns identified according to their context and subjects into reusable “Reference Content”. Consequently, the Enterprise Standards are the result of international subject matter experts and academic consensus. The Enterprise Standards has been developed in the following ways:
- Research and analyze the existing patterns in the organization.
- Identify common and repeatable patterns (the basis of the standards).
- Sort the repeatable patterns by:
- Best practices, enabling standardization and cost cutting.
- Industry practices, empowering performance for head to head competition.
- Leading practices, facilitating the innovation of value to develop differentiating capabilities.
- In order to increase the level of reusability and replication, package the identified patterns into Enterprise Standards.
- Build Industry accelerators within the standards, enabling organizations to adopt and reproduce the best practices, industry practices and leading practices.
Today, there are 136 different academic research subjects that have been packaged as reusable reference content. What is important is that they are both agnostic and vendor neutral, and are built on repeatable patterns that can be reused/replicated, and thereby implemented by any organization, both large and small, and regardless of its products, services and/or activities. (von Rosing & Laurier, 2015). All together, they describe the set of procedures an organization can follow within a specified area or subject in order to replicate the ability to identify, create and realize value, performance and standardization, etc.
The 136 different enterprise standards with their repeatable patterns have been categorized into 6 specific areas:
- Enterprise Management Standards with the official ID# LEAD-ES10EMaS.
- Enterprise Modelling Standards with the official ID# LEAD-ES20EMoS.
- Enterprise Engineering Standards with the official ID# LEAD-ES30EES.
- Enterprise Architecture Standards with the official ID# LEAD-ES40EAS.
- Enterprise Information & Technology Standards with the official ID# LEAD-ES50EITS.
- Enterprise Transformation & Innovation Standards with the official ID# LEAD-ES60ETIS.
References
- Alavi, M., & Leidner, D. E. (2001). REVIEW: KNOWLEDGE MANAGEMENT AND KNOWLEDGE MANAGEMENT SYSTEMS: CONCEPTUAL FOUNDATIONS AND RESEARCH ISSUES. [Article]. MIS Quarterly, 25(1), 107-136.
- Armstrong, J. S., & Brodie, R. J. (1994). Effects of portfolio planning methods on decision making: experimental results. International Journal in Marketing, 11(1), 73-84. doi: 10.1016/0167-8116(94)90035-3.
- Armstrong, J. S., & Green, K. C. (2007). Competitor-oriented Objectives: The Myth of Market INTERNATIONAL JOURNAL OF BUSINESS, 12(1), 115-134.
- Boston Consulting Group. (1970). Perspectives on experience. Boston, Mass.
- Bozeman, B. (2000). Technology transfer and public policy: a review of research and theory. Research Policy, 29(4–5), 627-655. doi: https://dx.doi.org/10.1016/S0048-7333(99)00093-1.
- Day, G. S. (1977). Diagnosing the Product Portfolio. Journal of Marketing, 41(2), 29-38.
- Elmaghraby, S. E. (1963). A NOTE ON THE EXPLOSION AND NETTING PROBLEMS IN THE PLANNING OF MATERIALS REQUIREMENTS. [Article]. Operations Research, 11(4), 530-535. doi: 10.1287/opre.11.4.530.
- Fitzgerald, A., & Inst Elect, E. (1992). ENTERPRISE RESOURCE PLANNING (ERP) – BREAKTHROUGH OR BUZZWORD (Vol. 359). Stevenage Herts: Inst Electrical Engineers.
- Gotel, O. C. Z., & Finkelstein, A. C. W. (1994, 18-22 Apr 1994). An analysis of the requirements traceability problem. Paper presented at the Requirements Engineering, 1994., Proceedings of the First International Conference on.
- Hevner, A. R., March, S. T., Jinsoo, P., & Ram, S. (2004). DESIGN SCIENCE IN INFORMATION SYSTEMS RESEARCH. MIS Quarterly, 28(1), 75-105.
- Ju, T. L., Yueh-Yang, C., Szu-Yuan, S., & Chang-Yao, W. (2006). RIGOR IN MIS SURVEY RESEARCH: IN SEARCH OF IDEAL SURVEY METHODOLOGICAL ATTRIBUTES. The Journal of Computer Information Systems, 47(2), 112-123.
- Kim, Y. S., & Cochran, D. S. (2000). Reviewing TRIZ from the perspective of Axiomatic Design. [Article]. Journal of Engineering Design, 11(1), 79-94.
- Kitchenham, B., Pearl Brereton, O., Budgen, D., Turner, M., Bailey, J., & Linkman, S. (2009). Systematic literature reviews in software engineering – A systematic literature review. Information and Software Technology, 51(1), 7-15.
- Laursen, K., & Salter, A. (2004). Searching high and low: what types of firms use universities as a source of innovation? Research Policy, 33(8), 1201-1215. doi: https://dx.doi.org/10.1016/j.respol.2004.07.004.
- March, S. T., & Smith, G. F. (1995). Design and natural science research on information technology. Decision Support Systems, 15(4), 251-266.
- Myers, M. D. (1997). Qualitative Research in Information Systems. [Article]. MIS Quarterly, 21(2), 241-242.
- Nerkar, A. (2003). Old Is Gold? The Value of Temporal Exploration in the Creation of New Knowledge. [Article]. Management Science, 49(2), 211-229.
- Newsted, P. R., Huff, S. L., & Munro, M. C. (1998). Survey Instruments in Information Systems. [Article]. MIS Quarterly, 22(4), 553-553.
- Nonaka, I., Umemoto, K., & Senoo, D. (1996). From information processing to knowledge creation: A Paradigm shift in business management. Technology in Society, 18(2), 203-218.
- Peffers, K., Tuunanen, T., Rothenberger, M. A., & Chatterjee, S. (2007). A design science research methodology for Information Systems Research. [Article]. Journal of Management Information Systems, 24(3), 45-77. doi: 10.2753/mis0742-1222240302.
- Perkmann, M., & Walsh, K. (2007). University–industry relationships and open innovation: Towards a research agenda. [Article]. International Journal of Management Reviews, 9(4), 259-280. doi: 10.1111/j.1468-2370.2007.00225.x.
- Ralph, P., & Wand, Y. (2009). A Proposal for a Formal Definition of the Design Concept. In K. Lyytinen, P. Loucopoulos, J. Mylopoulos & B. Robinson (Eds.), Design Requirements Engineering: A Ten-Year Perspective (Vol. 14, pp. 103-136): Springer Berlin Heidelberg.
- Rosenkopf, L., & Almeida, P. (2003). Overcoming Local Search Through Alliances and Mobility. [Article]. Management Science, 49(6), 751-766.
- Rosenkopf, L., & Nerkar, A. (2001). Beyond local search: Boundary-spanning, exploration, and impact in the optical disk industry. Strategic Management Journal, 22(4), 287-306.
- Sein, M. K., Henfridsson, O., Purao, S., Rossi, M., & Lindgren, R. (2011). Action design research. MIS Q., 35(1), 37-56.
- Slater, S. F., & Zwirlein, T. J. (1992). Shareholder Value and Investment Strategy Using the General Portfolio Model. Journal of Management, 18(4), 717-732. doi: 10.1177/014920639201800407.
- Stratton, R., & Mann, D. (2003). Systematic innovation and the underlying principles behind TRIZ and TOC. [Article; Proceedings Paper]. Journal of Materials Processing Technology, 139(1-3), 120-126. doi: 10.1016/s0924-0136(03)00192-4.
- Vincent, J. F. V., Bogatyreva, O. A., Bogatyrev, N. R., Bowyer, A., & Pahl, A.-K. (2006). Biomimetics: its practice and theory (Vol. 3).
- von Rosing, M., & Laurier, W. (2015, January-June). An Introduction to the Business Ontology. International Journal of Conceptual Structures and Smart Applications, 3, 20-42.
- von Rosing, M., Laurier, W., & Polovina, S. M. (2015a). The BPM Ontology. In M. v. R.-W. S. v. Scheel (Ed.), The Business Process Management Handbook (pp. 101-121). Boston: Morgan Kaufmann.
- von Rosing, M., Laurier, W., & Polovina, S. M. (2015b). The Value of Ontology. In M. v. R.-W. S. v. Scheel (Ed.), The Business Process Management Handbook (pp. 91-99). Boston: Morgan Kaufmann.
- von Rosing, M., Fullington, N., and Walker, J., Applying Ontology and Standards for Enterprise Innovation and Transformation of three leading Organizations, International Journal of Conceptual Structures and Smart Applications, Volume 4, 2016
- von Rosing, M., and Zachman, J. A., Using the Business Ontology to develop a Role Ontology, International Journal of Conceptual Structures and Smart Applications, Special Issue, Volume 5, 2016.
- Webster, J., & Watson, R. T. (2002). Analyzing the past to prepare for the future: writing a literature review. MIS Q., 26(2), xiii-xxiii.
- Wieringa, R., & Heerkens, H. (2008). Design Science, Engineering Science and Requirements Engineering Proceedings of the 16th IEEE International Requirements Engineering Conference (pp. 310-313). Los Alamitos: Ieee Computer Soc.
- Yamashina, H., Ito, T., & Kawada, H. (2002). Innovative product development process by integrating QFD and TRIZ. [Article]. International Journal of Production Research, 40(5), 1031-1050. doi: 10.1080/00207540110098490.